Bias in AI
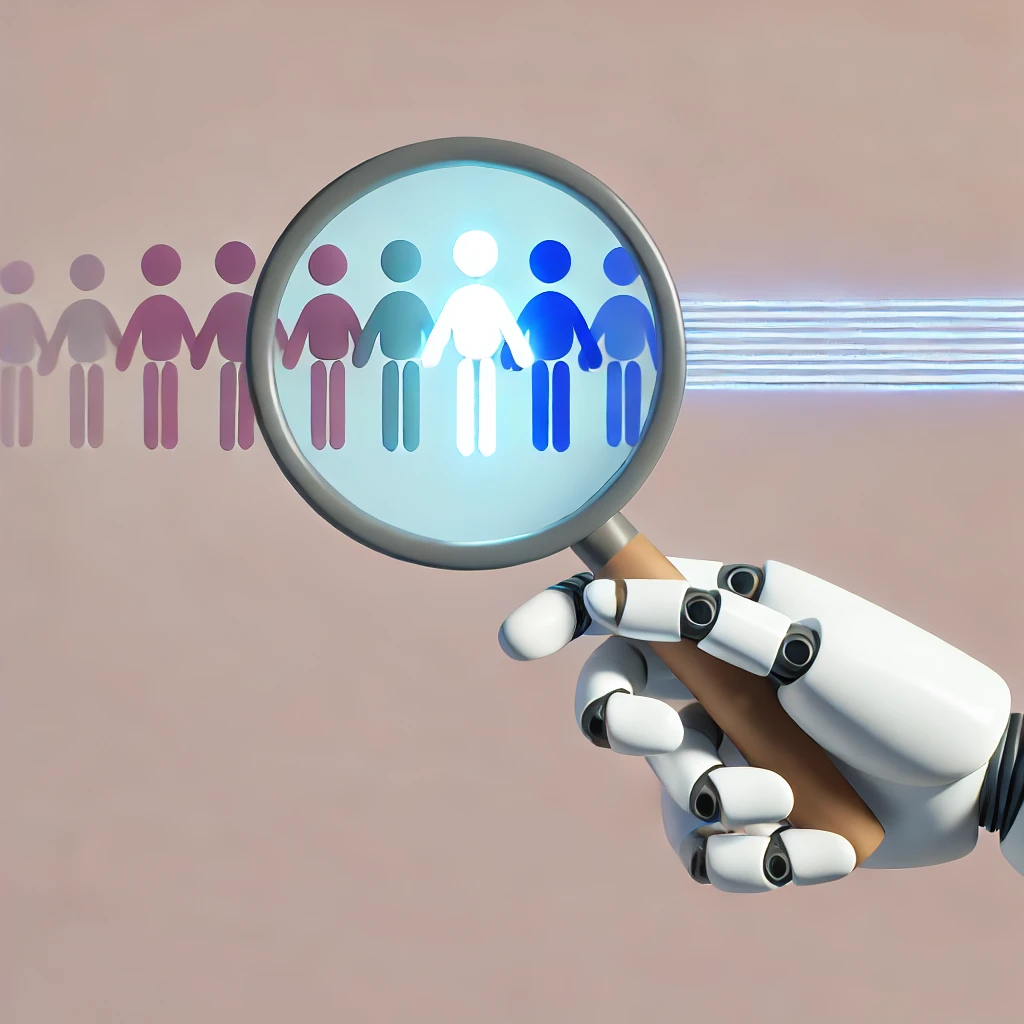
As AI algorithms become more integrated into beauty and skincare solutions, understanding and addressing bias is critical. From skin tone analysis to personalized recommendations, AI has the potential to either bridge or widen gaps in beauty inclusivity. In this section, we explore how bias in AI impacts beauty tech, and what companies are doing to address these challenges.
Types of Bias in AI
Training Data Bias
Training data bias occurs when the data used to train an AI system is not representative of the diversity of the real world. In beauty tech, this can result in AI systems that perform poorly for certain skin tones or types, leading to inaccurate product recommendations or analyses for underrepresented groups.
Algorithmic Bias
Algorithmic bias arises from the design of the algorithm itself. If the algorithm is not designed with inclusivity in mind, it may amplify biases present in the data or make biased predictions, affecting how different demographics are treated by beauty AI systems.
Racial and Gender Bias
Racial and gender bias in AI refers to AI systems that disproportionately favor or disadvantage certain racial or gender groups. In the beauty industry, this could manifest as AI-powered makeup or skincare tools failing to properly match products to darker skin tones or neglecting to consider diverse facial structures.
Case Studies and Examples
Pulse Oximeter Bias in Black Patients
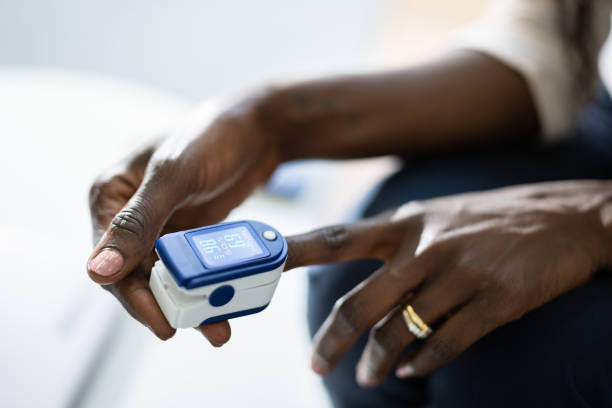
Pulse oximeters, vital for monitoring blood oxygen levels, have faced criticism for racial bias, with studies showing they are three times more likely to miss dangerously low oxygen levels in Black patients than in white patients. This causes delayed treatment and poorer health outcomes. While the FDA has introduced guidelines to improve testing diversity, they may still fall short of real-world conditions. Addressing this bias is essential for equitable healthcare.
Racial Disparities in Pain Assessment and AI Bias
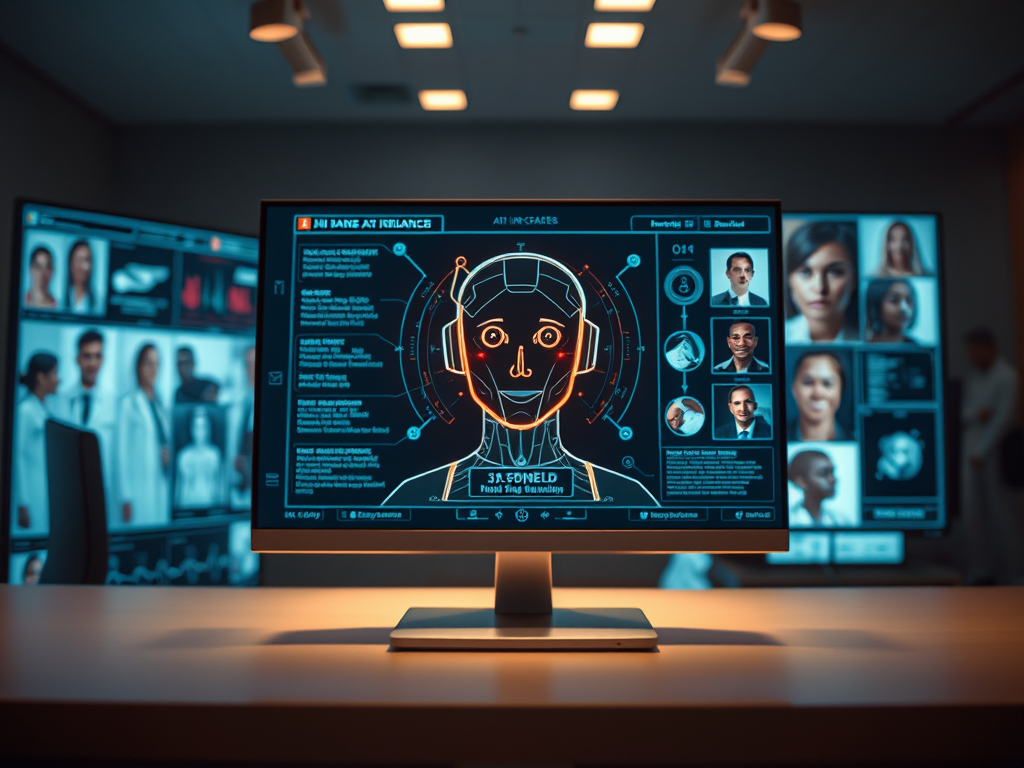
Dr. Adam Rodman’s study at Beth Israel Deaconess Medical Center found that AI chatbots, like Gemini Pro and GPT-4, perpetuate racial biases in pain assessment. Both AI models and human medical trainees consistently underassessed pain levels in Black patients, reflecting biases in the training data. This shows how AI systems can magnify human biases, leading to further inequalities in healthcare.
AI and Skin of Color Bias
By Dr. Jane Yoo
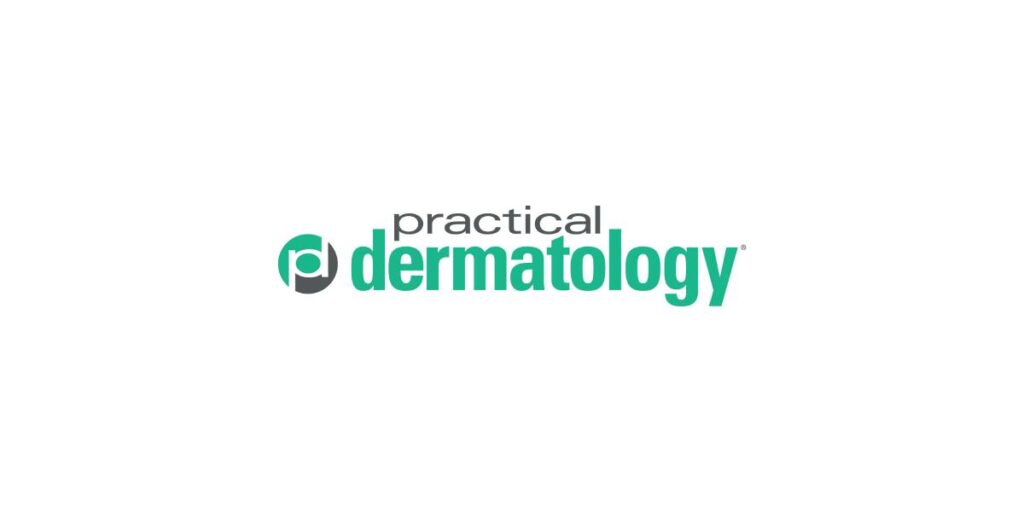
Facial recognition and dermatology AI tools have been shown to misclassify darker skin tones, leading to inaccuracies in diagnoses, particularly in detecting skin cancer. Studies from MIT revealed that major AI systems often underperform on darker-skinned individuals. Despite efforts like Google’s Monk Skin Tone scale, biases remain prevalent, showing the need for more inclusive data to improve AI performance and ensure equitable care across all skin types.
Dermatology AI and Skin Tone Bias
A Science Advances study found AI models for skin disease diagnosis perform worse on darker skin tones. Using the Diverse Dermatology Images (DDI) dataset, researchers found a significant performance gap between AI predictions for light and dark skin tones, particularly in detecting skin cancer. Fine-tuning these models on diverse datasets helped reduce this disparity, highlighting the importance of representative data to ensure reliable AI performance across all skin types.
Featured Resources
A Scoping Review of Reporting Gaps in FDA-Approved AI Medical Devices
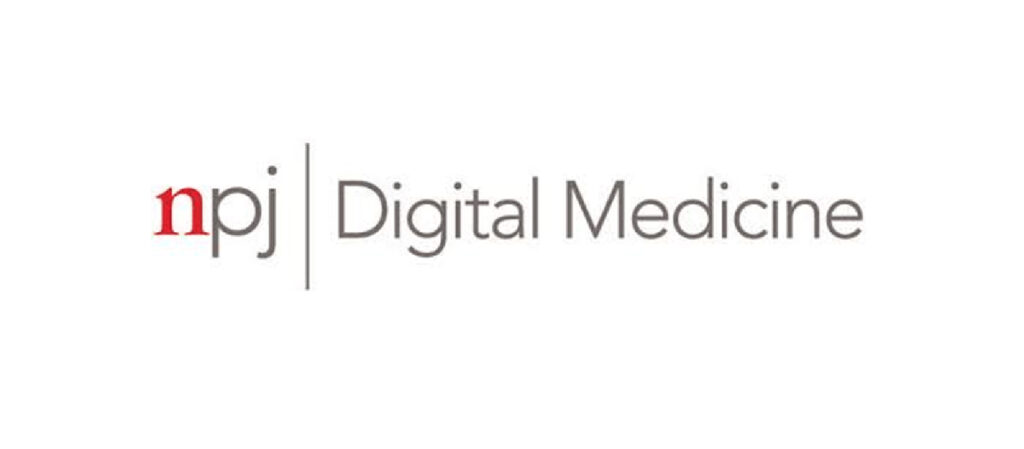
This review highlights transparency issues in FDA-approved AI medical devices, revealing that race, ethnicity, and socioeconomic data are rarely reported. With only 3.6% of devices reporting race/ethnicity and 99.1% providing no socioeconomic data, the lack of comprehensive demographic information increases the risk of algorithmic bias in healthcare. The findings emphasize the need for stricter regulatory frameworks to ensure AI devices are tested and validated across diverse populations.
Guide for Fair and Equitable AI in Health Care
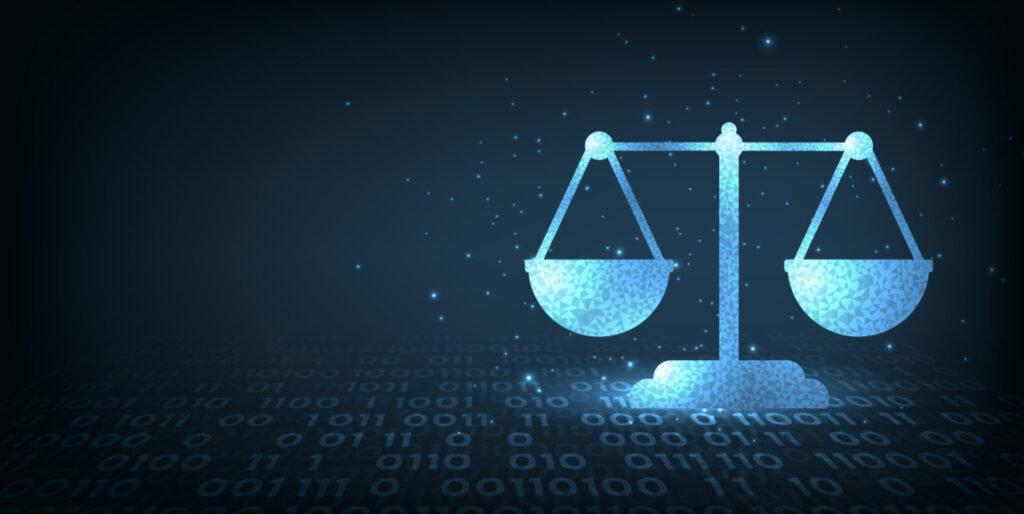
Healthcare systems have collected vast patient data through electronic health records, and AI now offers the potential to predict outcomes and improve care. Dr. Nigam Shah, Stanford Medicine’s chief data scientist, emphasizes the need for protocols to prevent bias in AI. Key questions include: Does the algorithm perform equally well for all demographics? Shah’s team is developing guidelines to ensure fairness, with algorithms undergoing fairness audits to verify consistent performance across diverse patient groups before clinical use.
Algorithmic Justice League
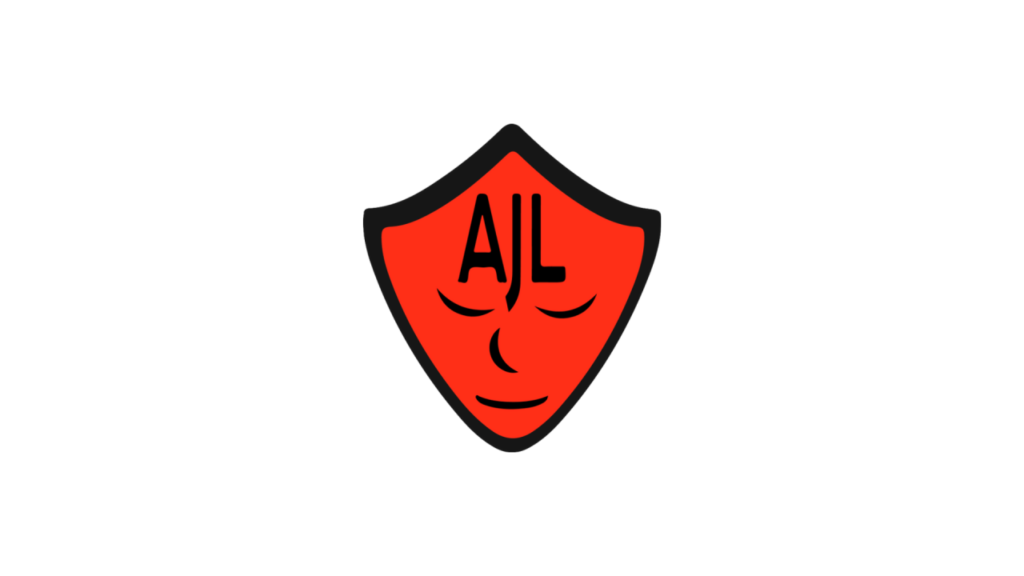
The Algorithmic Justice League (AJL) advocates for fair AI, highlighting how unregulated AI can reinforce discrimination in jobs, healthcare, and policing, and impact marginalized communities. AJL combines research, art, and advocacy to raise awareness, promote equitable AI, and support civil rights. With initiatives like #FreedomFlyers, AJL calls on individuals to protect biometric rights and urges policymakers and industry leaders to make AI accountable and fair for all.